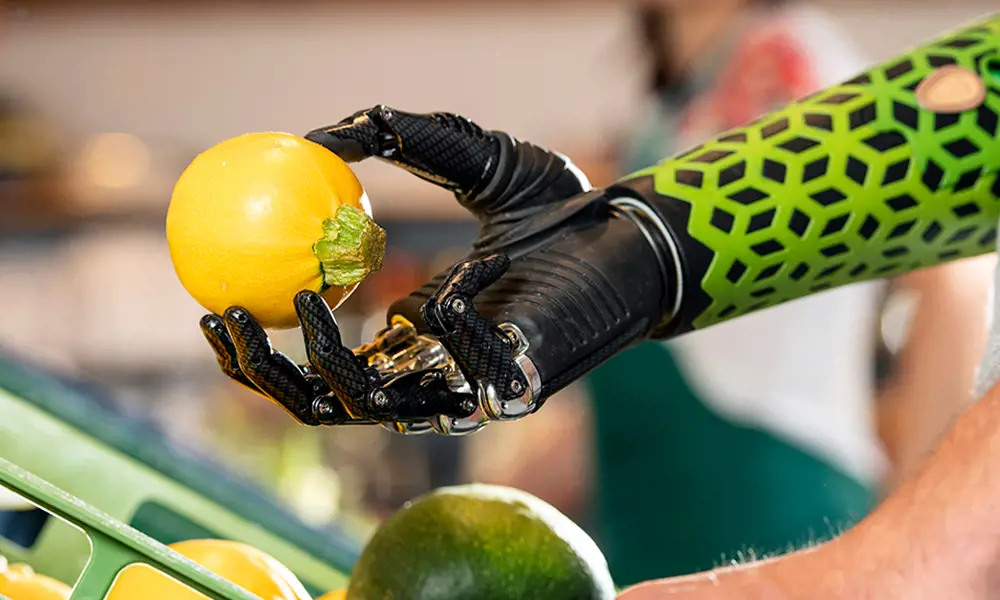
Machine learning boosts medical devices
💬 Shini says...
Ingenia 100 guest editor Dr Shini Somara tells us her thoughts
Biomedical technology has already come so far. Incorporating AI into these impressive technologies enhances their capabilities to greater levels of precision and efficiency. It’s exciting to learn how AI can increase bespoke medical care and therefore its effectiveness. And AI is allowing these innovations to be made available to us sooner.
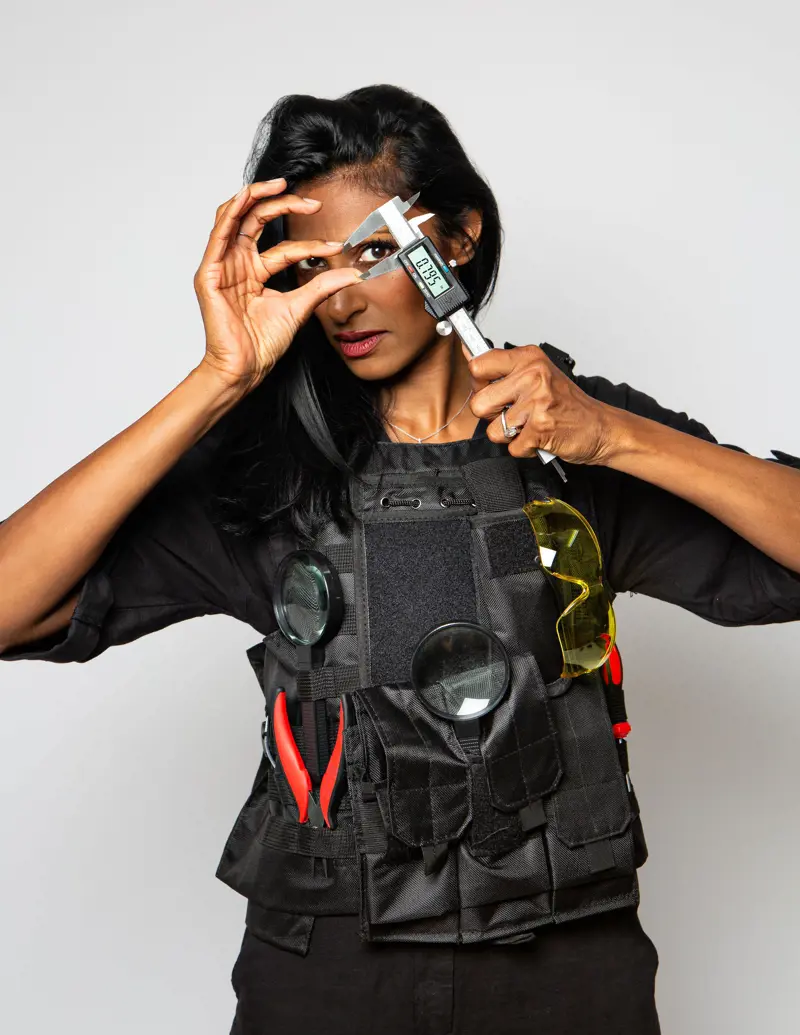
© Dr Shini Somara
Before generative AI and chatbots such as ChatGPT came onto the scene, other kinds of AI were quietly transforming all kinds of industries. In healthcare, bioengineers have been exploring how machine learning – a subset of AI – can improve medical devices and diagnostic tools, and even accelerate drug development.
In the last year, NHS trusts have developed several potentially lifesaving technologies based on machine learning. One system can analyse CT scans to improve cancer diagnosis and treatment, while another based on facial recognition can detect the quality of organs for transplant. Other equally important developments support doctors by preparing X-ray reports or reading blood test results, ultimately freeing up their time for more direct patient care.
Outside of the hospital, engineers are also exploring how machine learning can improve medical devices such as hearing aids and prosthetics. It could solve common complaints that people have with such devices, with the potential for noticeable improvements in day-to-day quality of life.
All about artificial intelligence
Do you know your neural networks from your LLMs? 👇
Artificial intelligence (AI) refers to a broad set of technologies that enable computer systems to perform tasks that would ordinarily require human intelligence, such as voice and facial recognition, analysing data and making decisions.
Machine learning is a subset of AI in which algorithms – “recipes” of mathematical operations – find patterns in large libraries of data. It can tirelessly sort through data around the clock to undertake tasks that humans would find tedious or onerous. Thanks to its pattern-spotting abilities, it is increasingly being used in healthcare, where huge databases, often gleaned from studies of many patients, can yield valuable insights.
Neural networks are types of machine learning models that mimic the human brain’s approach to processing data.
Generative AI is AI that can generate new content such as written material, imagery, videos or other data in response to prompts. To do this, it first studies massive libraries of training data for patterns and relationships using machine learning, before generating new content with similar characteristics.
Large language models (LLMs) are the machine learning models used for generative AI. For example, GPT-3.5 and GPT-4 are LLMs, available through chatbots such as OpenAI’s ChatGPT and Microsoft’s Copilot.
Hearing aids that mimic the brain
From adaptive noise cancellation in earbuds to voice recognition in smart speakers, many everyday technologies involve applying AI to sound. It’s no surprise, then, that hearing aid manufacturers use similar approaches to improve devices for wearers.
According to the Royal National Institute for Deaf People, 7 million people in the UK could benefit from hearing aids, but only about 2 million people use them. So, why the gap?
One of the major barriers stopping people wearing hearing aids is a lack of perceived benefit. For example, a big issue is distinguishing speech in loud environments such as in a crowded room or next to a busy road. Older devices tend to make everything louder, which is unhelpful for the listener and sometimes a downright unpleasant experience.
Today, medical device engineers integrate AI into hearing aids in the hopes of solving such problems. Starkey, based in Minnesota, has developed a range of hearing aids that selectively amplify conversation above background noise, even when that includes music with vocals.
To understand how this new generation of AI-enabled hearing aids work, it’s useful to look back over the devices’ history. The earliest form of electronic hearing aid was simply a microphone connected to an amplifier, which effectively turned up the volume on the signal it received and delivered this through a speaker in the wearer’s ear. The problem with this simplistic approach is that hearing loss is not uniform over all frequencies. Amplifying the entire signal amplifies noise, along with important components such as speech.

Starkey has incorporated its Genesis AI hardware into its invisible hearing aids (which are fitted in the ear canal and cannot be seen on the outside of the ear), as well as larger models of its hearing aids © Starkey
The next generation of hearing aids gave wearers more control, thanks to a tuneable chip called a digital signal processor (DSP). These chips convert the sounds captured by the hearing aid’s microphone into a digital signal that can be filtered or processed. With the DSP, audiologists could program the hearing aid to amplify specific frequencies. In practice, the audiologist fitting the device would test their patient’s hearing to determine which sound frequencies they could no longer hear and program the hearing aid to amplify only those.
“That technology has dominated for the last 10 to 15 years,” explains Achin Bhowmik, Starkey’s Chief Technology Officer. However, it didn’t help wearers surrounded by sounds with overlapping frequencies. Trying to clearly hear the people nearest to you in a busy restaurant is one example. It becomes even more difficult when there is music playing or if the room has echoes.
These scenarios cause problems because of the way that the brain processes sound. After sound is funnelled through the outer ear, it is initially processed in the inner ear before being sent to a part of the brain called the auditory cortex. Each of the roughly 100 million neurons in the auditory cortex are connected to tens of thousands of others. If a particular connection between neurons – called a synapse – is used a lot, it becomes stronger. This is what happens when we learn something new. Conversely, if it is used less, it weakens. Neuroscientists believe this is part of when we forget something – and is how the brain stays efficient.
This effect is at play when someone’s lived with hearing loss for a while. And according to Bhowmik, it is usually the case, as people tend not to immediately realise that they have hearing problems. “They sometimes find that when they get a hearing aid everything is louder, but they can’t understand it as well as they used to,” he explains. This is because the amount of signal being sent to the auditory cortex has decreased, weakening the synapses. The effect is particularly noticeable in situations where there is a lot of background noise. Unconsciously, we “forget” how to listen.
To help people distinguish the voice of who they’re talking to from background noise, most modern hearing aids preferentially amplify sounds coming from the direction the wearer is facing. They do this by having two microphones instead of one, which helps determine where sounds are coming from relative to the wearer. By comparing the strength of the signals received by the two microphones, it can selectively amplify sounds coming from directly in front of the user. For example, when both microphones receive a signal of equal strength, the sound is coming from the direction the wearer is facing (which is more likely to be something the wearer wants to pay attention to).
Starkey’s Genesis range of hearing aids adds an extra layer of AI processing to this to help the wearer to distinguish which parts of the signal they want to concentrate on and understand.
"[People] sometimes find that when they get a hearing aid everything is louder, but they can’t understand it as well as they used to,” Bhowmik explains. This is because the amount of signal being sent to the auditory cortex has decreased, weakening the synapses. Unconsciously, we “forget” how to listen.
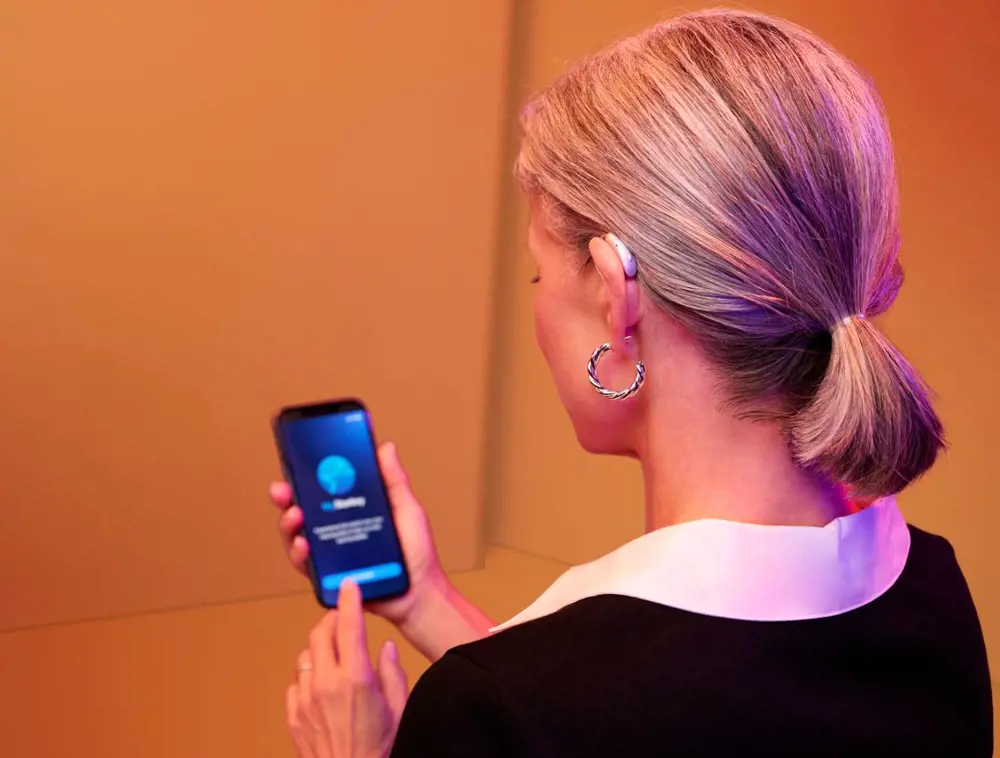
Starkey’s Genesis AI range of hearing aids link to a connected app, with which wearers can adjust settings, stream calls, and even translate languages and find lost hearing aids © Starkey
Central to this is a special type of processor chip designed specifically to run AI algorithms called neural networks. In neural networks, signals are treated like they are in the brain, says Bhowmik. When an artificial ‘neuron’ receives a signal, it processes it and sends the modified signal to other neurons. Just like with synapses, frequently used pathways become stronger, or in computer science parlance, their ‘weight’ increases. Lesser used pathways have a lower ‘weight’. This adjustment in weights is analogous to learning.
To determine the weight, the chip is trained with a variety of sounds, including speech and typical background noises in the same frequency range, like wind and the sound of machinery. As a result, it can pick out the exact frequencies of speech and amplify those in preference to non-speech frequencies. According to Bhowmik, this allows wearers to better distinguish sounds that fall in the same frequency range. This could not be done well with the previous DSP-based technology, he adds.
You might think that so much processing would render the hearing aids very power hungry. However, with many neurons – or rather, their electronic mimics – handling signals simultaneously, power consumption is reduced. In the case of the Genesis hearing aids, this means the devices can run for more than 50 hours on a full charge, twice as long as devices not using neural networks.
Another reason why the battery life is so long is to do with the chip’s physical structure. “For good battery life, it’s best to have the DSP and neural network all on one powerful chip,” Bhowmik explains. This is because all of the processing happens in one place, rather than it being shuttled back and forth between different units.
While this technology aims to directly mimic one of the ways in which the brain’s sensory systems works, other AI systems are fine-tuning how people can control prosthetic devices attached to their bodies.
Prosthetic arms that better adapt to wearers
According to a 2018 NHS review, about 55,000 people in the UK have limb differences. Those with upper limb differences might choose to wear a body-powered, passive or bionic prosthetic arm.
The majority of bionic prosthetic arms are myoelectric: controlled via electrical signals produced by contractions of the upper arm muscles. Typically, these signals are picked up through the skin by sensors in the socket of the prosthetic. This isn’t a new technology – it first appeared in prosthetic arm prototypes in the 1950s and 1960s – but still has a way to go before it works for every person with a limb difference, all the time.
To operate a myoelectric arm, individuals must learn new patterns of muscle contraction that will produce hand movements such as making a fist or rotating the hand. But the signals received by the prosthetic’s inbuilt sensors must always be the same for it to work consistently. In reality, the sensors can give different readouts over the course of a day for the same input from wearers. This can happen if the wearer’s residual limb swells, contracts or gets sweaty because of temperature changes as it can shift slightly in the socket. Signals can also change as the wearer gets tired.
Prosthetics manufacturer Ottobock, headquartered in Germany and Austria, hopes to solve these challenges. The company has developed a myoelectric prosthesis that uses AI to ensure that its performance is consistent throughout the day. Ottobock pairs the prosthetic with what it calls “MyoPlus control”, so that the hand’s fingers and thumb can hold objects in different ways. Since 2022, the NHS has supplied this AI-enabled prosthetic to people without a lower arm, whether they’d had an amputation or were born with a limb difference.
The signals received by a prosthetic’s inbuilt sensors must always be the same for it to work consistently. In reality, the sensors can give different readouts over the course of a day for the same input from wearers.
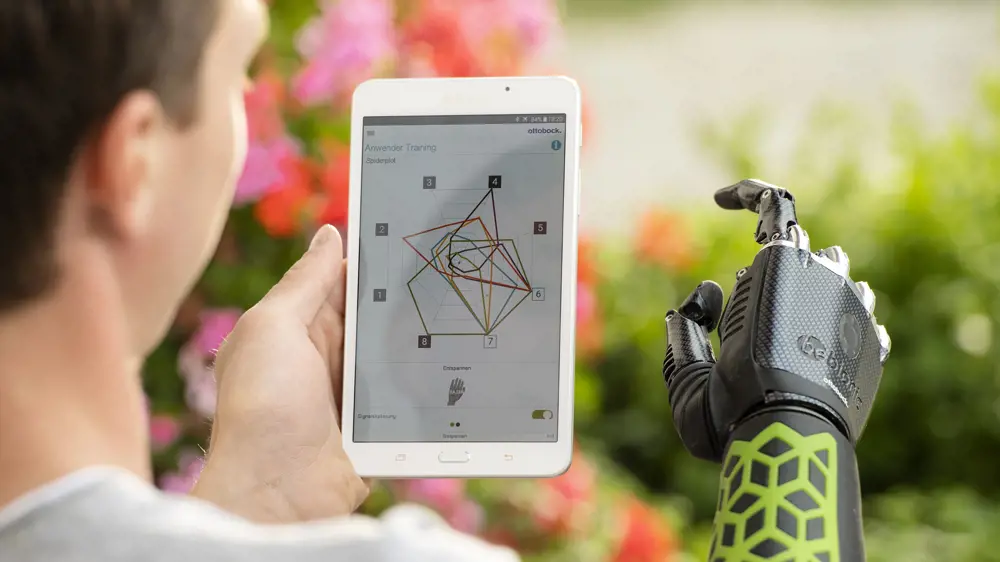
Wearers can configure Myo Plus pattern recognition with a smartphone or tablet © Ottobock
Martin Wehrle, a product manager at Ottobock, wears one of the prostheses because he was born with a limb difference. After a good night’s sleep, “I usually wake up super-energised, put on my arm and everything works fine,” explains Wehrle. “But if I’d been on a long flight or had a late night, it won’t work so well.” Over a day, depending on what he’s doing, “the arm’s performance starts to get a little worse.”
With previous non-AI enabled arms, Wehrle would have to work harder to contract the muscles in his residual arm to get the hand to make the movements he wanted. With the MyoPlus control arm, “it evens out differences in the myoelectric input, even if my skin is dry or it’s cold outside, which can have an effect, compared with how the signals are once my muscles have warmed up.” The arm also adapts to changes in signal if his muscles start to get tired or sweat starts to build up on his skin inside the prosthesis socket.
The key is a difference in the control protocols. “In a conventional myoelectric prosthesis, we assign a single function to each signal received by a skin sensor, such as open or close the hand, and those instructions are hard-coded into the controller,” explains Sebastian Amsüss, the arm’s lead designer. “The AI controller doesn’t follow hard-coded rules, but rather it adapts to the user.” The sensors in the socket detect signals from the wearer’s muscles and nerves and ‘show’ these to the AI system. The AI then uses algorithms to extract the information relevant to that individual that is needed for a specific prosthesis movement.
That “relevant information” comes when the person first receives the prosthetic and it is calibrated to them. “At the beginning, the system doesn’t know what to do,” explains Johannes Steininger, a software developer who worked on the project. For the calibration, the individual works with a prosthetics specialist who records the different signals that the sensors pick up from the skin of the wearer’s residual limb. “We record the different signals the user wants to use to open and close all the fingers, some of the fingertips to the thumb, or to rotate the hand. The AI is then trained to activate the motors inside the prosthesis to produce those movements,” he says.

Calibration of Ottobock’s myoelectric prosthetic hand takes place when an individual first receives the devices © Ottobock
This is very different from how a wearer would learn to use a non-AI prosthetic, Steininger adds. “There’s a big variability between how users’ muscles work and how their nerve signals look, and whereas before [the wearers would] have to be trained to produce the sort of signals the control system could recognise, now we capture the specific signal pattern of the individual and use that to train the system.”
Similarly to how neural pathways can get weaker for a person with hearing loss, amputees who do not begin to use myoelectric prostheses soon after limb loss can lose the pathways involved in hand movement. AI can help to prevent this, Amsüss says. This is because it allows the wearer to continue using the same muscle and nerve pathways as before the amputation, rather than having to learn new patterns. As a result, the synapses remain strong. The closer the pathways are to the ones operating before the limb was lost, the better they are preserved, he says. “Using pre-amputation nerve signals is much more effective than conventional control strategies. This positively affects both the motor and the sensory cortex.”
For somebody who has lost a limb, operating a prosthetic by the same parts of the brain that controlled the limb before injury has another important advantage. Rather than feeling that it is “like a brick attached to their arm” – a comparison that Amsüss says some amputees have made – the approach can help them to feel as if the prosthetic is more a part of their body and incorporate it into their mental image of themselves.
We are still a very long way from artificial intelligence replacing human brains, if indeed we ever get to that point. But AI is proving its worth in mimicking some of the ways that our brains function, and using this ability to restore some functions or senses that people might have lost.
Contributors
Achintya K. (Achin) Bhowmik is the Chief Technology Officer and Executive Vice President of Engineering at Starkey. He leads Starkey’s efforts to transform hearing aids into multifunctional health and communication devices with advanced sensors and artificial intelligence technologies.
Johannes Steininger is an embedded software developer at Ottobock Vienna and currently lead engineer of the MyoPlus pattern recognition and its successor products. Before joining Ottobock in 2017, he studied biomedical engineering at TU Graz with a focus on machine learning and signal processing.
Martin Wehrle has been Global Product Manager for Upper Limb products at Ottobock Vienna since 2012. Before that, he was a technical trainer at the Ottobock Academy after graduating in electrical engineering from the University of Applied Sciences in Kempten i. Allgäu.
Sebastian Amsüss is a System Engineer and Development Lead at Ottobock in Vienna. In his role, he is the main developer of hand prostheses and is responsible for system integration and interfaces. Previously, he wrote his dissertation on ‘Robust electromyography-based control of multifunctional prostheses of the upper extremity`.
Keep up-to-date with Ingenia for free
SubscribeRelated content
Health & medical

A gamechanger in retinal scanning
2006 MacRobert Award winner Optos rapidly became a leading medical technology company and its scanners have taken millions of retinal images worldwide. There is even a display at the Science Museum featuring the Optos development. Alastair Atkinson, of the award-winning team, describes the personal tragedy that was the trigger for the creation of Optos.
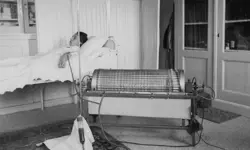
Kidney dialysis
Small haemodialysis machines have been developed that will allow more people to treat themselves at home. The SC+ system that has been developed is lighter, smaller and easier to use than existing machines.
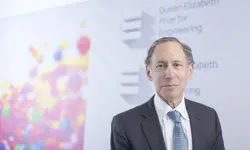
Engineering polymath wins major award
The 2015 Queen Elizabeth Prize for Engineering has been awarded to the ground-breaking chemical engineer Dr Robert Langer FREng for his revolutionary advances and leadership in engineering at the interface between chemistry and medicine.
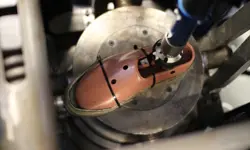
Blast mitigation and injury treatment
The Royal British Legion Centre for Blast Injury Studies is a world-renowned research facility based at Imperial College London. Its director, Professor Anthony Bull FREng, explains how a multidisciplinary team is helping protect, treat and rehabilitate people who are exposed to explosive forces.
Other content from Ingenia
Quick read
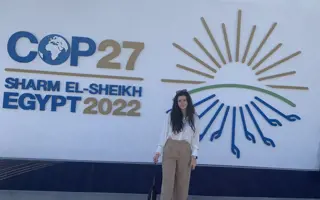
- Environment & sustainability
- Opinion
A young engineer’s perspective on the good, the bad and the ugly of COP27
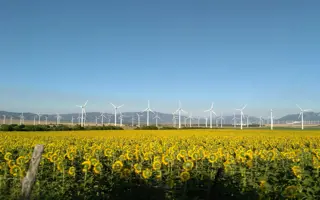
- Environment & sustainability
- Issue 95
How do we pay for net zero technologies?
Quick read
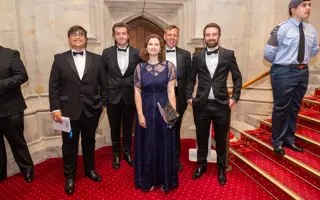
- Transport
- Mechanical
- How I got here
Electrifying trains and STEMAZING outreach
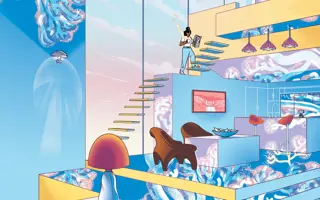
- Civil & structural
- Environment & sustainability
- Issue 95