
Facial Recognition
Facial Recognition
Many facial biometrics-based systems have been deployed over recent years but with varying success – variability of lighting conditions often leads to unreliable performance. Dr Thomas Heseltine, a biometrics consultant for Aurora Computer Services and Dr Nick Whitehead, Airport Developments Programme Manager at Atkins, describe the technology behind a new approach that resolves these problems using infra-red instead of visible light images.
Post-September 11, myriad US airports installed biometric systems in a bid to protect against and reassure travellers and employees about potential terrorist threats. But the facial recognition systems trialled at numerous airports, including Logan airport in Boston, Oakland International Airport in California and TF Green Airport in Providence, were not a success. Failure to identify a high percentage of passengers raised question marks over the validity of the technology.
Nearly a decade later, security systems developed by Atkins are being rolled out that promise to improve on this reputation. These systems use facial recognition software developed by Aurora Computer Services. But rather than performing facial recognition using visible light images, as had taken place in previous systems, these systems use infra-red images. In recent trials conducted with thousands of people, no-one was falsely rejected, that is, incorrectly turned away by the system, nor were there any false acceptance events where the wrong person was allowed to proceed. This was achieved by improved system design and the advances made by the development of infra-red facial recognition. So what exactly is the technology and why choose infra-red?
Biometrics are the digital analysis – using cameras or scanners – of biological characteristics to match profiles to databases of people. Facial recognition as well as finger print readers, retina and iris scanners are all examples of reliable biometric technologies. However, many in the biometrics industry believe that face recognition is the most promising technique for busy transport terminals as it is deemed more acceptable to the general public (see Which biometric is best?).
Rather than using fingerprint scanners or other devices that require people to touch, click or stand in an exact position, facial recognition systems use relatively ‘distant’ cameras, and therefore, are less intrusive. After extensive trials this has led to higher levels of public acceptance than other forms of biometric – an important factor in any deployment of such security systems.
Face matching
The latest facial recognition technology works by using software that can pick a person’s face from a crowded image, extract that face from its surroundings and compare it to a database of stored images. To do this, the software uses algorithms that first localise a face and then measure various features of that face for recognition.
This process, known as face matching, consists of several steps. Face detection identifies the extremities of the ‘interesting’ part of the image and then eye detection marks the centre points of the eye socket, providing fixed reference points for other measurements. Facial landmarks, describing facial elements from the size, shape and locality of a feature to minute details of skin texture, are also identified.
These features are mapped into a statistical model that can distinguish between different people and also compensate for reasonable variations in expression and head angle. Biometric templates are generated and compared to those in a database, to provide a final decision, or in most cases a ‘likeness score’, that indicates the probability of the two matching.
There are two requirements needed for face matching to take place reliably. The image under analysis must first be of sufficiently high resolution so the algorithms can measure its features accurately. And second, the picture must be taken under similar lighting conditions to the existing image in the database, to minimise differences in the appearance of skin tone, texture and facial features (see figure 1). If either of these criteria are not met, the recognition system will make more false rejections.
Resolution issues can be solved by using a high resolution camera to ensure an image contains enough pixels for accurate analysis. However controlling lighting conditions is more problematic. While software developers have written algorithms that can compensate for differences in lighting that may alter the database and verification images, recognition systems have still struggled to accurately make the match.
So what does this mean in practice? In an airport, for example, how does using facial recognition software help to verify its passengers? As false rejection rates increase, so do boarding queues. In an attempt to maintain a steady flow of passengers from the departure gate to the plane, facial recognition systems have been enclosed in kiosks or booths so that lighting can be controlled when the images are taken. However, this approach can be considered as intrusive and is becoming increasingly impractical with today’s preference for open plan airports.
Clearly a new solution is needed.
Infra-red facial recognition
To overcome the problem of lighting in facial recognition systems, biometrics researchers at Aurora turned to infra-red facial recognition, where a camera and flash system operates with infra-red light rather than visible light (see figure 2). To date, researchers worldwide have typically considered one of two options; thermal infra-red or near infra-red.
A thermal infra-red system uses sensors to measure the emitted heat from the object in question. The advantages of this method are that thermal images can be obtained under every lighting condition and are fairly robust to differences in facial expressions. However the images produced via thermal infra-red vary depending on the body temperature of the subject, which can be easily distorted by weather and recent exercise.
Instead, the researchers looked at near infra-red. The near infra-red bandwidth lies in the reflective part of the infra-red spectrum, falling between the visible light band and thermal infra-red band. Crucially, this portion of the infra-red band is invisible to human eyes, making near infra-red illumination unobtrusive when used in a facial recognition system.
Importantly, the near infra-red images are recognisable by humans, so an image could still be double-checked by a security officer in the event of a false rejection. In addition, a near infra-red system would be cheaper than the thermal equivalent.
With this in mind, researchers at Aurora went on to develop a camera and flash system that operates with near infra-red light (figure 3). The system consists of a dual enrolment/verification unit that contains two image cameras and two infra-red light sources secured into an adjustable base.
Each camera has a wide angle lens that ensures subjects (including wheelchair users) from 97 cm to 213 cm tall can be photographed without having to stoop or stand in an awkward position. The infra-red light sources provide consistently illuminated images, regardless of ambient lighting conditions, and produce images with better depth, gradient and shape information. Researchers also developed an infra-red-sensitive camera, bringing together a wish-list of features into a single face recognition device. This included an infra-red CMOS sensor with global shutter, intelligent flash control and fast on-board processing power to provide a very high level of fine grain control at rapid frame rates.
The system operates by first detecting a passenger’s face and eyes (figure 4). These are then aligned to form a so-called ‘normalised’ token image. This image is assessed for quality, and rejected if any features fall outside the system requirements. For example, a subject might be wearing glasses that obscure their face or they may sneeze. Alternatively, parts of the image could be ‘noisy’, dark or blurred. Information from this quality assessment routine is then fed back to the camera, allowing for real-time dynamic compensation.
With the token image accepted, facial algorithms then model variations in facial expression, head angle and appearance, compensating for disparities that could lead to a false rejection.
Aurora’s algorithms, developed over several years, work by using a machine-learning technique. The system is supplied with thousands of examples of infra-red face images, which are analysed to create a mathematical model of facial variation. The model attempts to identify and extract natural variations between different images of the same person (known as ‘within class variation’) in order to compensate for head angle (pose) and expression. Given the infra-red technology, the model does not need to compensate for variations in lighting, which vastly simplifies this problem.
The learning algorithm also attempts to identify characteristic features that can discriminate between individuals (known as ‘between class variation’). This learning process takes weeks of intensive computation distributed across a network of multi-core processors. The discriminating features that can be identified range from minute details of small scale texture and shading, to large scale face shape, structure and spatial locality
of features.
With the mathematical model, complete face-matching can take place. Faces are compared by minimising any ‘within class variance’, while simultaneously maximising the ‘between class variance’ in order to make a classification decision. The biometric template generated from this actual system consists of only 6.3to 31.5 Kb of data and describes the statistical variance of the face without storing any image data. In a security system a first template would be produced at biometric enrolment, and a second generated at a verification point. These can be compared to produce a match score in just 4 microseconds.
Real-life systems
Trials have shown the system takes, on average, just 4.7 seconds to verify a person’s identity, including the time taken to instruct and position the individual. However, 80% of those people were verified in less than two seconds and work is already underway to further improve the system. Right now, researchers are extending facial matching algorithms to match a person with their e-passport.
The latest e-passports contain a colour image of the owner encoded on an integrated chip, and Aurora researchers have recently developed an engine that can validate this image against a live infra-red image of the person presenting their e-passport at the security desk. In a similar way to the fast-flyer programmes already in operation across airports worldwide, self-service check-ins could soon be available for mass passenger transit.
Keep up-to-date with Ingenia for free
SubscribeOther content from Ingenia
Quick read
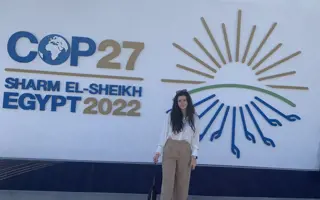
- Environment & sustainability
- Opinion
A young engineer’s perspective on the good, the bad and the ugly of COP27
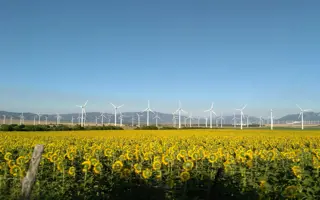
- Environment & sustainability
- Issue 95
How do we pay for net zero technologies?
Quick read
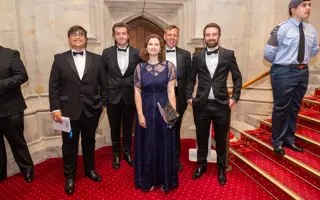
- Transport
- Mechanical
- How I got here
Electrifying trains and STEMAZING outreach
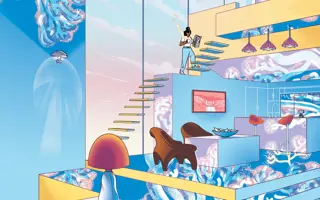
- Civil & structural
- Environment & sustainability
- Issue 95