
AI shakes up the world of weather forecasting
Did you know?
❄️ The intricate science of weather forecasting
- Traditional weather forecasts take hours to generate on supercomputers that consume as much energy as thousands of homes
- Forecasts from weather agencies such as the UK’s Met Office don’t just go to weather apps – they’re critical for the aviation and defence sectors
- Data from weather stations, balloons, buoys and satellites all over the world informs the models they use
The weather is governed by deeply complex physics. And yet, within seconds of swiping open a weather app, we can see a reasonably accurate forecast for the next couple of weeks. On a mundane level, forecasting might support a decision to wear a raincoat or pack an umbrella; in extreme cases, it warns residents in the path of a storm or hurricane to flee.
Forecasts are underpinned by precisely defined equations that attempt to describe the chaos of weather physics. Into these equations goes data continuously gathered from weather balloons, weather stations and satellites around the world. Processing all this data are supercomputers, each of which may consume as much energy as thousands of homes. For once, AI holds a surprisingly less computationally intensive – and less energy-intensive – answer. That’s because once it’s been trained, it consumes a fraction of the compute power of the massive models run in conventional weather forecasting.
Google DeepMind’s AI weather forecasting model, GraphCast, drew widespread acclaim when it was published in late 2023 in the journal Science. While not the first of its kind, DeepMind’s research showed the model outperformed the gold standard of medium-range weather prediction in 90% of measures. And where traditional weather forecasting methods take over an hour to generate – and require a supercomputer to do so – GraphCast can produce a 10-day forecast in 45 seconds.
For this, GraphCast won the 2024 MacRobert Award, the UK’s most prestigious prize for UK engineering innovation, presented by the Royal Academy of Engineering. “It’s a massive technological leap forward in weather forecasting,” said Dr Steve Allpress FREng, who was on the judging panel. “It really is very impressive.”
Once GraphCast has been trained, it consumes a fraction of the compute power of the massive models run in conventional weather forecasting.
So fast, so good
GraphCast is among a stable of AI technologies developed at Google DeepMind since its inception in 2010. The company has built a reputation for designing AI systems to solve challenges across the board in science and engineering. Most famously, in October 2024, DeepMind’s CEO and Co-Founder, Sir Demis Hassabis FREng shared the Nobel Prize in Chemistry with Professors John Jumper and David Baker for their work demystifying and creating new proteins. Hassabis, Jumper and their team had decoded a decades-old biological problem, predicting how proteins fold up into 3D shapes. These structures give us vital insights into diseases and help us design better drugs. Google DeepMind’s AI system AlphaFold, released in 2021, predicts protein structures from their amino acid sequences. Scientists worldwide now use AlphaFold to help them study malaria vaccines, and treatments for Parkinson’s and Alzheimer’s, with more medical challenges on the way.
But if there’s one prediction problem people have been puzzling over a lot longer than protein folding, it’s weather forecasting. Today, for the lab behind so many AI breakthroughs, GraphCast is one of its proudest projects, aligning with a core DeepMind tenet to build AI systems that benefit everyone. “The weather affects everything,” says Andrew El-Kadi, a research engineer at DeepMind who works on the team that developed GraphCast. “From deciding whether to take an umbrella with you, to potentially life-altering situations like cyclones and extreme temperatures.”
“If we can improve the lead time [in which] we can issue warnings for individuals and get them out of harm’s way when extreme weather is coming, that’s going to save lives,” said Dr Peter Battaglia, head of the GraphCast team and weather group, at the Royal Academy of Engineering’s Innovation Incoming event in October. “We’re only going to see an increase in the importance of this as climate changes become more extreme.” Events unfolding a matter of days later underscored the significance of these words, as according to Reuters, early warnings of the catastrophic floods in Valencia went ignored by the regional government, leading to hundreds of deaths.
Computing chaos
How does conventional weather forecasting work? 🖥️
By far the dominant approach to weather forecasting today is numerical weather prediction (NWP), where meteorologists create models on supercomputers that describe the way the atmosphere changes. The UK Met Office uses a Cray XC40, which is so powerful it can perform 14,000,000,000,000,000 (14 quadrillion) sums per second.
The Cray XC40 and supercomputers like it rely on data – and a lot of it. Each day, the Met Office receives about half a million data points, including temperature, humidity, and wind speed and direction. It is gathered by sensors in the atmosphere, on land and the ocean surface, and on satellites.
With this data as a starting point, the supercomputer then creates a model of the atmosphere. It fills in the gaps where no data is available, choosing the pattern that most closely matches the data. This is called data assimilation and is “the most complex and expensive part of forecasting”, according to the Royal Meteorological Society. Then, the prediction is made: a mathematical model based on physics equations that factor in how air in the atmosphere behaves, as well as the Earth’s rotation and the transitions of water into vapour, liquid and ice. Meteorologists attach a confidence level based on probability. They also continually check that the forecast is on track, adjusting it if it’s not.
Conveying risks can be a matter of life or death in the case of extreme heat, flooding or a tornado – or even something as simple as needing to grit roads because of icy temperatures. In extreme scenarios, weather experts and other professionals pool their expertise to make decisions. So, despite supercomputers, advanced satellites and AI, we still need human weather forecasters. Their qualities are difficult to replicate: real-world experience and the ability to make observations and connections in a way that algorithms cannot.
Fluid beginnings
GraphCast came into being as one step in a longer evolutionary process for DeepMind. Ever the champions of AI in fundamental science, the company was exploring how it could simulate complex physical systems, such as fluids, molecules, astrophysical systems, and power grids. “DeepMind has always had an eye on trying to use machine learning to do physical simulations,” says El-Kadi.
Six years ago, Battaglia was leading a team applying AI systems called graph neural networks (GNNs) to modelling the movement of fluids. GNNs learn relationships between data points in both time and space, after being trained on vast amounts of historical data. It was a natural next step to apply the same techniques used for modelling fluids to weather systems – or essentially, how fluids such as water and gases in the atmosphere move over time. “That’s really where, two and half years ago, things started turning towards weather,” says El-Kadi.
DeepMind’s foray into the weather began with a local precipitation prediction system trained with radar data, called NowCasting, before the company branched out to weather on a global scale. To find training data for this, it turned to the European Centre for Medium-range Weather Forecasts (ECMWF), home to the world’s largest archive of numerical weather prediction data. DeepMind trained its models with four decades of weather information from ECMWF’s high-quality data set, ERA5.
Conventional weather forecasting, such as NWP (see ‘Computing chaos’), involves expert meteorologists writing equations that capture the complex physics of weather, which are then solved by supercomputers. Using machine learning, GraphCast builds a time evolution of atmospheric data and learns statistical relationships between the dataset, and what will happen next.
Training costs a lot of energy, but once that’s done, AI weather models such as GraphCast can be run very easily – a key benefit. “AI gets lots of criticism for the energy it’s using across all the different large language models, but believe me, traditional weather forecasting uses vast amounts of compute and energy,” said Professor Penelope Endersby CBE FREng, CEO of the Met Office, who was also on the Innovation Incoming event panel. “That is the big advantage, much more than any accuracy gain.”
To unlock this potential, powerful hardware would be needed. “To train these models, we need very specific hardware that is very good at doing the types of maths involved in machine learning,” says El-Kadi. “In house at Google, we have our TPUs.” The TPU, or tensor processing unit, is a Google-invented chip used for tasks such as machine learning.
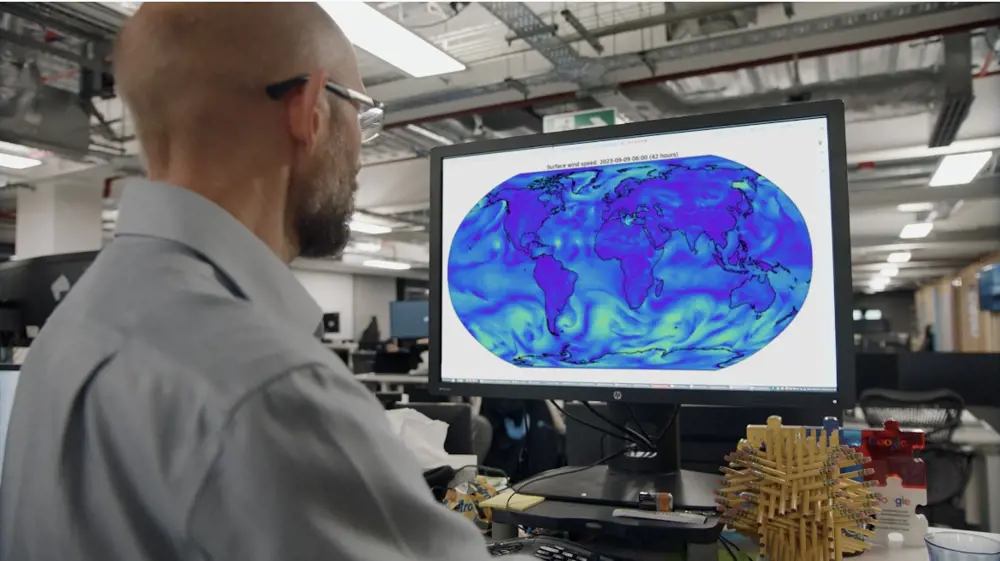
GraphCast’s forecasts cover over a million grid points representing the entire surface of Earth © Google DeepMind
In the last four years, these chips have advanced rapidly, making it possible for this hardware to handle monumental amounts of data. Each forecast is made over a million grid points representing the entire surface of the Earth. At each grid point, GraphCast predicts what different variables will be at the Earth’s surface and across 37 levels of altitude in the atmosphere. It predicts all of these four times a day for a 10-day forecast, explains El-Kadi. “I think you can see how this adds up to a lot of data.”
With so many possible scenarios on the cards, AI needs to be trained to learn how to forecast the weather. To do this, it’s asked to predict the weather on two consecutive timesteps (each six hours apart) in the past. “Then we say, you were right here, wrong there. Try again,” says El-Kadi. This process, called loss calculation and back propagation, tweaks the model’s parameters so it can make better predictions of what the actual weather was in the second step, he says.
GraphCast then takes it a step further, looking at how different points in the atmosphere influence one another. “If you know a cold storm is happening on France’s north coast, it’s likely that in six to 12 hours’ time that storm is going to affect the south coast of England,” says El-Kadi. “Weather patterns are quite local, and the cold storm will probably move north.” So, they tell the model that the weather on the Earth’s surface affects the weather in other places. This is where the GNNs, which excel at learning from spatially structured data, come into their own, as GraphCast then spots connections between points on Earth, to learn how they affect each other when it comes to forecasting the weather.
Black swans and hurricanes
GraphCast’s acid test was in September 2023, when Hurricane Lee, a massive tropical cyclone, was heading to the east coast of the US and Canada. Nine days before it made landfall, GraphCast accurately forecast it would hit Nova Scotia. Official forecasts had been hedging on it landing on major northeast cities or missing them altogether.
More accurate hurricane-path forecasting would be a huge advantage for disaster relief. Weather data, coupled with information about where people live, is vital for rescue, explained catastrophe risk modelling expert Dr Joshua Macabuag FREng, also speaking on the Innovation Incoming panel. Macabuag was deployed to the Caribbean in 2017 when Hurricanes Harvey, Irma and Maria passed through within three weeks of one another. He and his colleagues didn’t know where the area with greatest need would be at first. “If you end up on the wrong island, it could take three or four days to get to the correct one,” he said.
Saving lives isn’t the only benefit of GraphCast’s accuracy. Macabuag is now Co-Founder of Renew Risk, which applies risk modelling to renewable energy. Extreme weather, such as tropical storms and major hurricanes, pose a significant risk to offshore wind farms. Macabuag explained that having greater certainty that potential damages are on the way gives operators time to check control systems, make sure they’re protected or stop operations, if necessary.
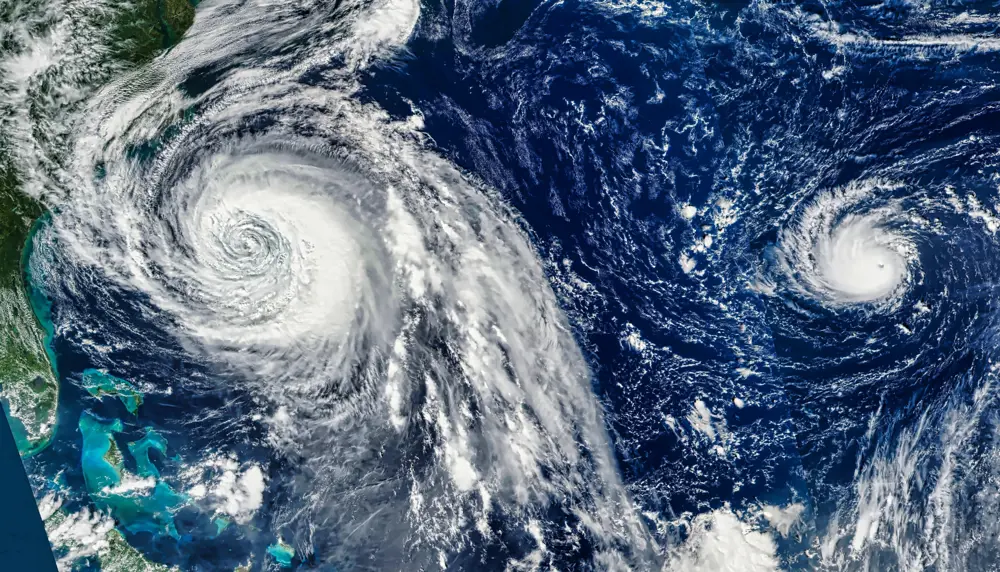
Satellite images of Hurricanes Lee and Maria above the Atlantic Ocean in 2023 © Shutterstock
You might ask, if it’s trained on historic data, how can GraphCast forecast extreme weather events unlike anything we’ve seen before – so-called ‘black swan’ events? And can GraphCast adjust for climate change causing more unpredictable weather patterns than ever? “I don’t know whether we can predict more intense winds than have ever been recorded in the ERA5 dataset,” said Battaglia. But, he added, when this does happen in real life, it’s important to ensure the learning methods are incredibly efficient, so they can predict them well.
El-Kadi explains that, as it continues to learn from recent weather events, GraphCast can predict the weather more accurately than any other model. This means it can better predict extreme weather, too. “We are constantly updating it as new data comes in,” says El-Kadi. “So, it is learning from the impacts of climate change and severe weather and is better able to spot patterns and apply that going into the future.”
Meteorologists in the loop
GraphCast has already had an outsized impact. “I was blown away by how quickly it had been brought to being extraordinarily effective. It’s an astonishing example of what engineering can do,” said Professor Sir Richard Friend FREng FRS, who chaired the MacRobert Award Committee that chose GraphCast as the 2024 winner.
Because it is open-source technology, other scientists around the world can use it and tailor it to their needs. Weather agencies are making 10-day forecasts with GraphCast, and it is being run live as an experiment by ECMWF. “You can actually go and see the output on their website,” El-Kadi enthuses. Currently, ECMWF is also using GraphCast to inform internal decisions on which warnings to output and send to local governments. The GraphCast team constantly liaises with weather agencies such as the Met Office, which receives GraphCast’s outputs; and almost all countries in Europe are members of ECMWF.
So, scientists aiming to understand and predict the Earth’s atmospheric phenomena will very much remain in the loop, says El-Kadi. “It’s important we’re still liaising with expert meteorologists, and that the model is being used to inform policymakers, rather than bypassing their decisions.”
At Innovation Incoming, Endersby was animated about the opportunities that AI presents for weather forecasting but reckoned we’re “nowhere near” a model that can do everything numerical models can. She cautioned that, before weather agencies can rely on them for everything, we need to be certain that they’re as trustworthy as traditional NWP. “The level of trust you’d need for the app in your pocket that tells you whether you’re going to have a picnic or hang out your washing is somewhat different to the level of trust for when we’re routing your plane across the Atlantic or telling you where a nuclear dispersion cloud might go,” she said. Meteorologists will run AI models and experiment with them, but only alongside NWP. “Unfortunately, I think I’m going to be riding two horses for quite some time yet.”
The level of trust you’d need for the app in your pocket that tells you whether you’re going to have a picnic or hang out your washing is somewhat different to the level of trust for when we’re routing your plane across the Atlantic or telling you where a nuclear dispersion cloud might go.
Professor Penelope Endersby FREng, CEO of the Met Office
In a way, the meteorological community is not alone in this. DeepMind published work on a hybrid model in July, which is partly physics-based and partly AI-based. Unlike GraphCast, and like conventional weather models, the hybrid model presents a set of forecasts to capture the inherent uncertainty. It forecasts not only weather, but also longer-term climate metrics over decades.
It’s another tool in our arsenal to help us monitor climate change, and reaffirms DeepMind’s commitment to its north star. So, too, did the company’s recognition from the MacRobert Award judges. “[It] helps serve as a compass for us in a very profound way,” said Battaglia, in October. “It says, what you’ve done here in applying AI to weather was good for society … Keep going, do more of that, bring more people into this mission.”
***
Quotes from Dr Peter Battaglia, Professor Penelope Endersby CBE FREng, Sir Richard Friend FREng, and Dr Joshua Macabuag FREng were taken from the Royal Academy of Engineering’s panel discussion Innovation Incoming – MacRobert Award special, ‘Weather warnings from AI’
Nominations for the 2025 MacRobert Award are open until 31 January 2025.
Contributors
Andrew El-Kadi is a research engineer at Google DeepMind, working on the Weather effort. He completed his MEng in mathematics and computer science at Imperial College London in 2023. His current work focuses on scaling and operationalising machine learning weather models.
Keep up-to-date with Ingenia for free
SubscribeRelated content
Environment & sustainability
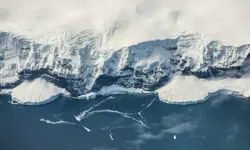
The extreme engineering behind life on an Antarctic station
Antarctica is one of the most challenging environments on the planet, but of enormous scientific importance to humanity. Technology and engineering is essential to enable the British Antarctic Survey's researchers to pursue their science effectively in this awe-inspiring landscape.

Recycling household waste
The percentage of waste recycled in the UK has risen rapidly over the past 20 years, thanks to breakthroughs in the way waste is processed. Find out about what happens to household waste and recent technological developments in the UK.
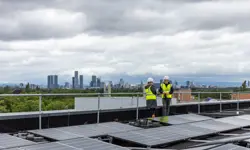
Upgrade existing buildings to reduce emissions
Much of the UK’s existing buildings predate modern energy standards. Patrick Bellew of Atelier Ten, a company that pioneered environmental innovations, suggests that a National Infrastructure Project is needed to tackle waste and inefficiency.
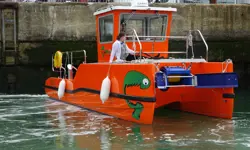
An appetite for oil
The Gobbler boat’s compact and lightweight dimensions coupled with complex oil-skimming technology provide a safer and more effective way of containing and cleaning up oil spills, both in harbour and at sea.
Other content from Ingenia
Quick read
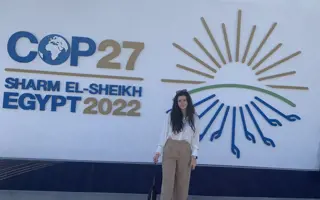
- Environment & sustainability
- Opinion
A young engineer’s perspective on the good, the bad and the ugly of COP27
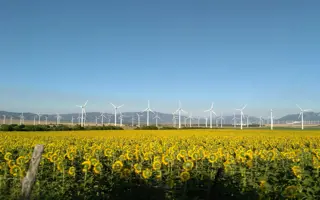
- Environment & sustainability
- Issue 95
How do we pay for net zero technologies?
Quick read
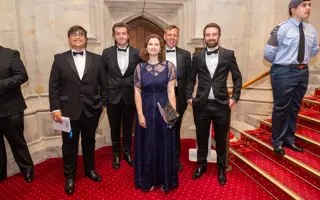
- Transport
- Mechanical
- How I got here
Electrifying trains and STEMAZING outreach
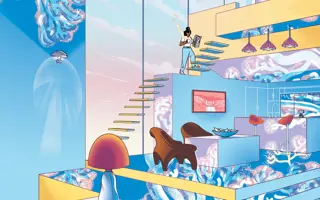
- Civil & structural
- Environment & sustainability
- Issue 95