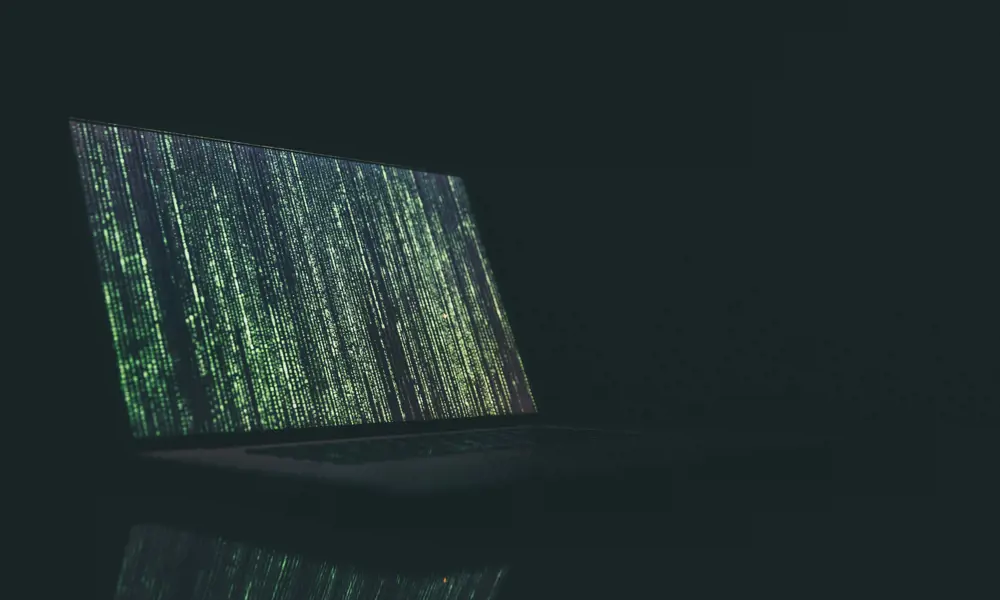
AI is not magic but it is complex
Artificial Intelligence (AI) is software that uses data to make decisions. The controversy surrounding it comes with its complexity. It typically operates with many sources of data, using complex logic that is hard for a human to understand or explain.
The digitisation of core infrastructure and services opens up many new opportunities for the use of AI in all aspects of our daily lives. As engineers we will be faced with the question of whether it is ethical to use AI in the systems we build, and our reputations will be won or lost on the future outcomes of these decisions.
In the Statement for Ethical Principles, updated by the Royal Academy of Engineering and the Engineering Council in 2017, there were four fundamental principles to adhere to. These came under the categories: honesty and integrity; accuracy and rigour; leadership and communication; and respect for life, law, the environment and public good. These principles provide a solid foundation on which to build new engineering practices.
As engineers we will be faced with the question of whether it is ethical to use AI in the systems we build, and our reputations will be won or lost on the future outcomes of these decisions
Technologies in general are not inherently ethical or unethical. It is the use that we put them to that determines whether a particular engineering system is ethical or not. AI is no exception, but as an engineer considering embedding AI into a system, it is necessary to step a little deeper into the technology to understand where the ethical risks occur.
AI is not a single technology. It is an assembly of technologies. At its centre is the AI decision-making logic, which works on an optimised store of knowledge based on the data that it has been exposed to. This decision-making logic is fed data about the current status and then produces a result that is acted upon in some way. If it is in an actively learning mode, then the result, and the outcome or change in the situation, is fed back into the decision-making technology to improve its knowledge store and hence the accuracy of future decisions. Around the decision-making logic are the components that capture and assemble the input data and act on the output decisions. Any one of these surrounding components can have as much effect on the outcome of the AI systems as the decision-making logic at the core.
An AI project team must determine the impact and the scope of that impact on the individuals and society affected by the AI system’s decisions. This will establish the level of quality that is needed in the decision-making process and any additional support that the system must provide.
For example, consider the use of AI to choose which travel destination to feature on a travel booking website. The impact of a bad choice on the individual using that website is usually minimal and short term. However, what about a scenario where AI is assisting a doctor in a medical diagnosis or assessing people for jobs or mortgages? Any resulting decision is likely to have a significant and long-term effect on the individual under consideration.
With this focus in place it is possible to specify the minimum level of accuracy that is required. This will then decide how to validate results, the explanation and consent needed from the individual, the circumstances under which a human decision-maker needs to be involved, and any mitigation management that needs to be in place if the decision is wrong.
Once the necessary accuracy of the decision-making is determined, the team need to consider what data can be practically (and legally) fed into the decision-making process when the system is running. This will include the scope, accuracy, precision and access speed of the data. This may be stored data or data that is being generated live within the system (such as data from sensors). We then need to decide whether this data is sufficient to drive the accuracy needed.
AI must be trained with real-world data. It is important that the profile of this data matches the profile of data that is to be fed into the system when it is live. Without this, the results may be biased or just plain wrong. It is the responsibility of the engineering team to verify the training data whether they are training the AI themselves or bringing in a decision-making model from a third party.
It is the responsibility of the engineering team to verify the training data whether they are training the AI themselves or bringing in a decision-making model from a third party.
AI technologies operate in a pipeline where raw data supplied to them is transformed into a format that is efficient for the decision-making logic. This transformation may reduce the precision or filter values from the incoming data and hence be making decisions on a smaller set of data than is expected. If, for example, in the case of image recognition, the transformations are lowering the definition of the incoming images to speed up the decision logic, it has to be determined whether this will materially impact the quality of the results below the bar needed by the rest of the system.
Since the world is constantly changing, so is the data that is produced. Whether the AI model is using feedback from its decisions to dynamically improve its accuracy or if it is operating in a consistent mode, it will need constant monitoring and updates to correct any bias or obsolescence that may be creeping in.
Finally, data from the system may be fed into other systems. This secondary usage may be planned and managed, or unplanned due to leakage or theft of data. The engineering team has a duty of care to safeguard the system components and data flowing through it, to ensure it cannot be raided or misdirected by third parties.
Much of this activity is already considered and carried out for complex physical components in engineering projects. AI is not magic, but it is complex. As engineers, we need to apply and evolve the standards we use for complexity in the physical world for the digital world. We need to ensure that our digital systems are operating in the ethical way that was intended.
To make great engines you need quality parts. AI algorithms are the engines of the future
To underpin the use of AI in engineering we need clarity from government or law on data ownership. This is especially important when new data is derived from multiple sources and the scope of liability when it comes to data supplied for decisions. This will help to clarify responsibility for a correct result and it may evolve though regulation or best practice.
Open standards for data contracts and their associated terms and conditions are critical as the basis for automatic enforcement and auditability. This needs to be a part of an open metadata and governance ecosystem that supports and manages digital systems.
There is also a need for standard data set definitions representing the minimum requirements for data that is used for specific type of decision. For example, there are standards for the type of aluminium that can be used for building aircraft – so there should also be standards for the quality and content of weather and other type of environmental data used by air traffic management.
It is time to take the engineering of data in the digital world as seriously as we take the use of raw materials in the physical world. To make great engines you need quality parts. AI algorithms are the engines of the future – so let’s get focused on the standards and methods that will ensure they deliver on their promise.
***
This article has been adapted from "AI is not magic but it is complex", which originally appeared in the print edition of Ingenia 80 (September 2019).
Contributors
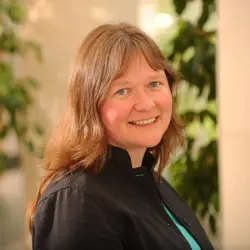
Mandy Chessell CBE FREng is the Founder of Pragmatic Data Research, which is a consultancy focused on accelerating digital transformations through innovative data architectures and governance. At the time of writing, Mary was an IBM Distinguished Engineer, Master Inventor and Fellow of the Royal Academy of Engineering. She leads the ODPi Egeria open source project and is a trusted advisor to executives from large organisations, working with them to develop their strategy and architecture relating to the governance, integration, and management of information.
Keep up-to-date with Ingenia for free
SubscribeRelated content
Technology & robotics

When will cars drive themselves?
There are many claims made about the progress of autonomous vehicles and their imminent arrival on UK roads. What progress has been made and how have measures that have already been implemented increased automation?

Autonomous systems
The Royal Academy of Engineering hosted an event on Innovation in Autonomous Systems, focusing on the potential of autonomous systems to transform industry and business and the evolving relationship between people and technology.

Hydroacoustics
Useful for scientists, search and rescue operations and military forces, the size, range and orientation of an object underneath the surface of the sea can be determined by active and passive sonar devices. Find out how they are used to generate information about underwater objects.

Instilling robots with lifelong learning
In the basement of an ageing red-brick Oxford college, a team of engineers is changing the shape of robot autonomy. Professor Paul Newman FREng explained to Michael Kenward how he came to lead the Oxford Mobile Robotics Group and why the time is right for a revolution in autonomous technologies.
Other content from Ingenia
Quick read
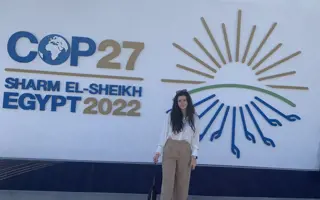
- Environment & sustainability
- Opinion
A young engineer’s perspective on the good, the bad and the ugly of COP27
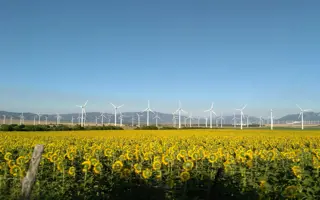
- Environment & sustainability
- Issue 95
How do we pay for net zero technologies?
Quick read
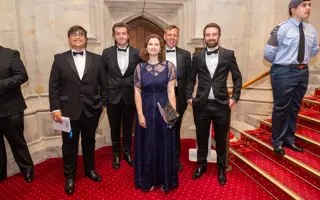
- Transport
- Mechanical
- How I got here
Electrifying trains and STEMAZING outreach
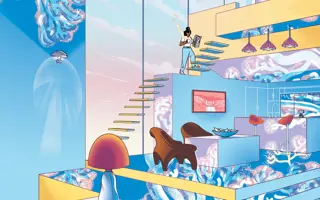
- Civil & structural
- Environment & sustainability
- Issue 95