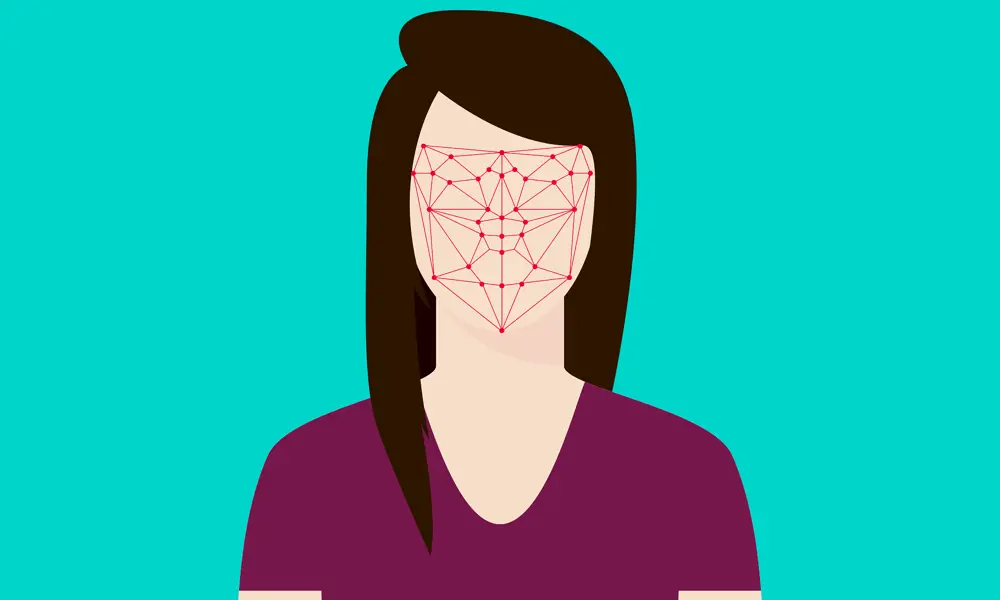
3D facial recognition technology
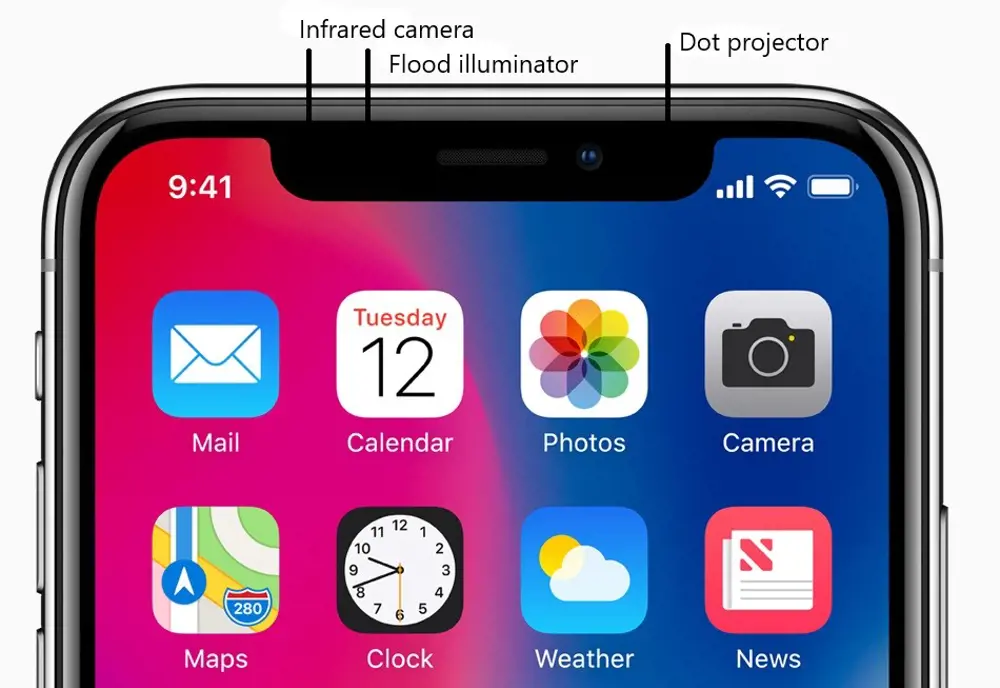
The iPhone X’s 3D facial recognition technology projects over 30,000 dots onto a user’s face to map their features and uses the information to unlock the phone in the future. The dots are invisible to the human eye but can be seen through an infrared camera, which captures the image and sends the data for confirmation. The flood illuminator is an invisible infrared light that identifies facial features even when it’s dark © Apple
There are several techniques in use for facial recognition but, essentially, it is a two-step process: feature extraction and selection, followed by classification. Many traditional processes use algorithms to identify facial features that are then used to search for other images with matching features.
Recently there has been increased interest in using 3D techniques for facial recognition. To understand this increased attention, we first need to understand how a task that all of us perform thousands of times a day, quickly matching faces we see to ones we know, presents such a challenge for a machine.
The first challenge comes from the ‘sheep effect’: the tendency of all sheep to look the same to you and me, while appearing quite distinctive to each other and the shepherd. Our vision is more accurate – sheep are all pretty much the same – but the shepherd’s vision is clearly much more useful. Much the same applies to our own faces, which are really all very similar (as seen by sheep perhaps but ‘really’ too) but seem usefully very distinctive to us. However, differences in face shape are quite modest and can be confused by things such as glasses and changing facial shape in expressions that we easily discount. Machines find recognising such changes more difficult, which is why, for example, people are asked to look expressionless in passport photos that will be used for recognition by machines.
So, what has changed? Much of the interest is just the impact of ever-increasing, faster and less energy consuming, processing power being available. This makes the considerable data processing requirement for facial recognition in a device such as a smartphone more practical. The same effect has also driven the wide application of speech recognition, although this needs even more processing power and uses communications and remote ‘cloud’ processing. But an even bigger gain has come from using better data, particularly a 3D image of your face rather than just an ordinary picture. In this respect, the machine is using better data than us; although, of course, we also see in 3D using our two eyes this is probably not so important for recognising people.
For a machine such as a smartphone, a 3D image of a face is much simpler to match reliably and this also solves problems with recognition when your face is slightly turned away, for example. Anyone with a recent iPhone or some gaming consoles will know that this also works in many lighting conditions, even in the dark, which is a problem for some other facial recognition technologies. This is typically done by ‘structured lighting’: the device projects a particular pattern of invisible infrared dots onto your face from several separated sources and analyses the video image these present. This reveals a 3D shape in much the same way as the angled shadows from a Venetian blind falling across a complex object. The information is then used to identify distinctive features on the surface of a face, such as the contour of the eye sockets, nose and chin. Although there is also much interest in laser radar (or ‘lidar’) for 3D imaging, for example for self-driving cars, it is currently far too expensive and not accurate enough to use for 3D identification of faces. However, the structured lighting approach, combined with faster processing and some extra techniques for ignoring hats and glasses for example, works well – although it is still imperfect and most likely not as good as humans. It is still probably the best of the biometric ID techniques, but the accuracy of recognition of 2D faces in sources such as CCTV feeds still needs much improvement. Some gaming consoles also successfully use similar techniques to analyse whole body movements. Expect the results to get better and be used ever-more-widely in the future as processing power and data capture improve further.
***
This article has been adapted from "How does that work? 3D facial recognition technology", which originally appeared in the print edition of Ingenia 79 (June 2019).
Keep up-to-date with Ingenia for free
SubscribeRelated content
Technology & robotics

When will cars drive themselves?
There are many claims made about the progress of autonomous vehicles and their imminent arrival on UK roads. What progress has been made and how have measures that have already been implemented increased automation?

Autonomous systems
The Royal Academy of Engineering hosted an event on Innovation in Autonomous Systems, focusing on the potential of autonomous systems to transform industry and business and the evolving relationship between people and technology.

Hydroacoustics
Useful for scientists, search and rescue operations and military forces, the size, range and orientation of an object underneath the surface of the sea can be determined by active and passive sonar devices. Find out how they are used to generate information about underwater objects.

Instilling robots with lifelong learning
In the basement of an ageing red-brick Oxford college, a team of engineers is changing the shape of robot autonomy. Professor Paul Newman FREng explained to Michael Kenward how he came to lead the Oxford Mobile Robotics Group and why the time is right for a revolution in autonomous technologies.
Other content from Ingenia
Quick read
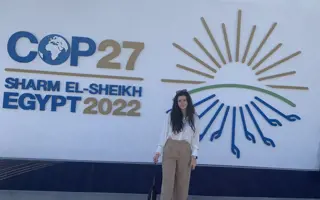
- Environment & sustainability
- Opinion
A young engineer’s perspective on the good, the bad and the ugly of COP27
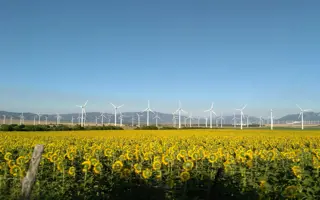
- Environment & sustainability
- Issue 95
How do we pay for net zero technologies?
Quick read
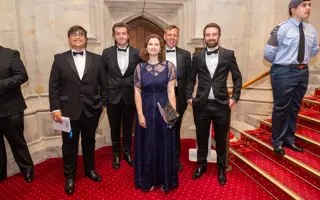
- Transport
- Mechanical
- How I got here
Electrifying trains and STEMAZING outreach
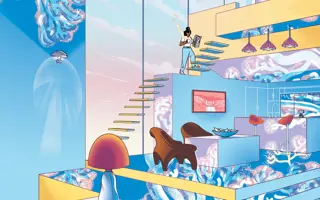
- Civil & structural
- Environment & sustainability
- Issue 95